SMART DIGITAL WATER TECHNOLOGY
Research Themes: Software Technology & Intellligent Systems, Water & Maritime
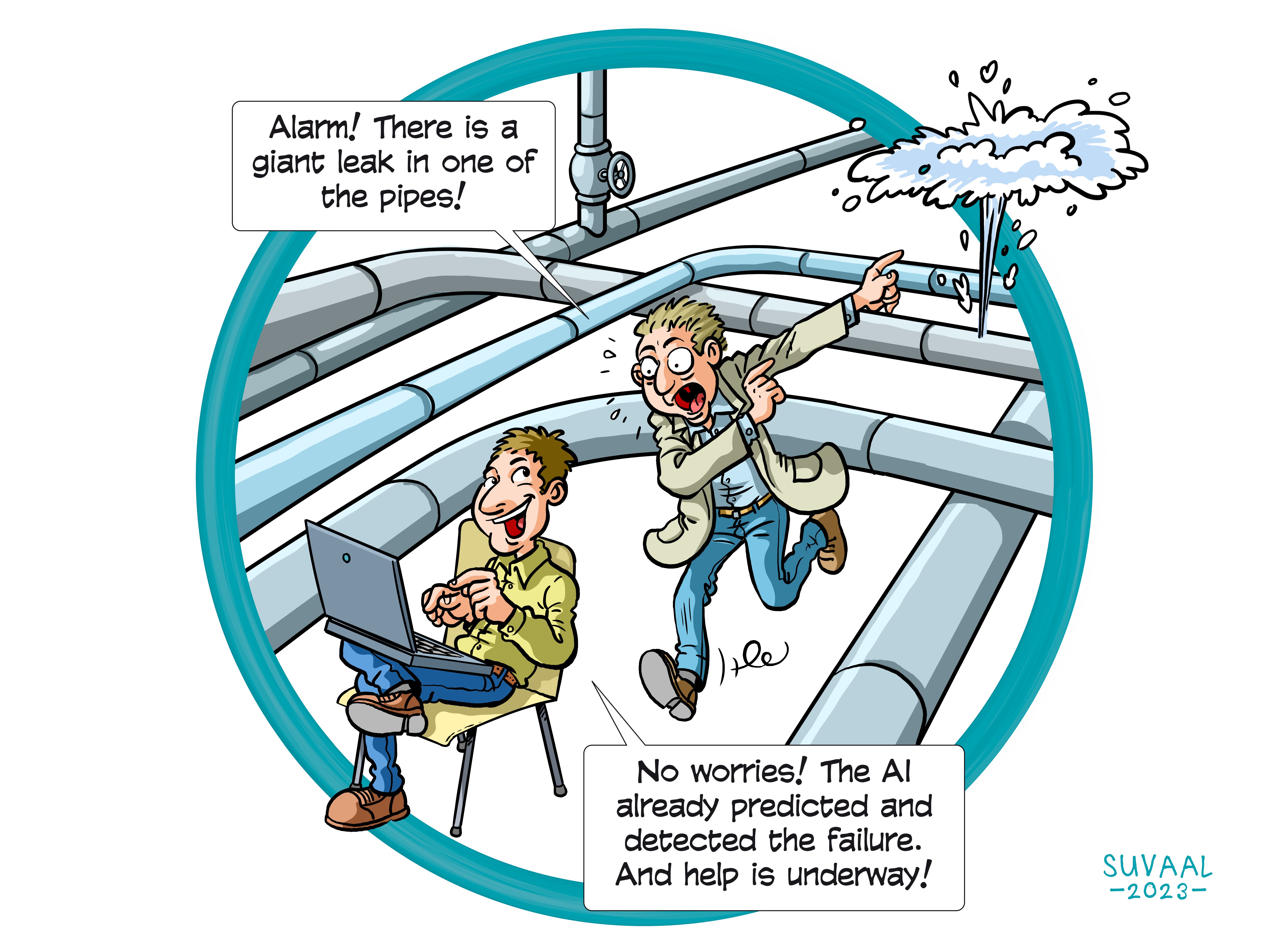

A TRL is a measure to indicate the matureness of a developing technology. When an innovative idea is discovered it is often not directly suitable for application. Usually such novel idea is subjected to further experimentation, testing and prototyping before it can be implemented. The image below shows how to read TRL’s to categorise the innovative ideas.
Summary of the project
By implementing AI techniques Prof. Kapelan aims to improve our urban water infrastructure, both drinking water distribution and urban drainage systems, by doing things in a smarter way. With his research he hopes to contribute to the challenges such as climate change and increasing population and urbanization. The researcher has developed / is developing Artificial Intelligence (AI) based methods that can be used to improve different aspects of operation, maintenance or long term planning of urban water infrastructure. These methods help to better understand the network performance, improve the utilization of various resources (water, energy) and predict or detect various system failures, ultimately resulting in improved asset management.
For instance, using data from pressure and flow sensors already installed in the drinking water distribution network, he has developed a real time, AI-based technology that detects pipe bursts/leaks, equipment and other failures. Where normally in the past someone had to take action based on visual identification of leaks – water emerging through the ground - the current system raises an automated alarm when such an event is detected from the corresponding changes in observed pressure and flow signals. Artificial neural networks are used to predict the pressure and flow values for the near future assuming no events and then combined with Bayesian networks to convert the discrepancies obtained between the predicted and observed into an alarm. Similar methods have been developed to detect water quality events in drinking water system (e.g. discoloration events), blockages, collapses and various structural sewer faults in urban drainage networks and process failures at water treatment plants.
Another example of a digital water technology is the methodology for real time control of drainage systems aiming to minimize the overflow of our combined sewer system into our surface water. When there is too much water in the urban drainage system – due to heavy rainfall for instance – a safety feature is to release the overflow into our canals and rivers resulting in a pollution event. With smart real time control of pumps and other devices combined with sensor data he aims to minimize such overflows. The challenge here is to take into the account the unpredictable rainfall. A similar approach is used for reducing nitrogen emissions at wastewater treatment plants. The AI-based model is first developed to predict accurately the level of emissions at the plant. This is then combined with suitable control measures to reduce the emission levels.
Despite the above successes in development of novel AI-based methods and technologies for urban water infrastructure, their implementation in a rather risk-averse water sector is still lagging behind. This remains a challenge to be addressed in the future.
What's next?
The researcher will continue to develop smarter ways to improve our urban water infrastructure, finding novel ways to address different challenges. The next step is moving away from more traditional smart water technologies to develop another type of smarter solutions in the context of circular economy. The researcher is currently co-developing one such solution where resources that are normally discarded are abstracted from several places across the urban water cycle resulting in a creation of new bio composite materials with wide ranging applications.
With or Into AI?
With or Into
Prof. Dr. Zoran Kapelan
Waternet, KWR, NPSP, UK water utilities
Faculties involved
- CEG