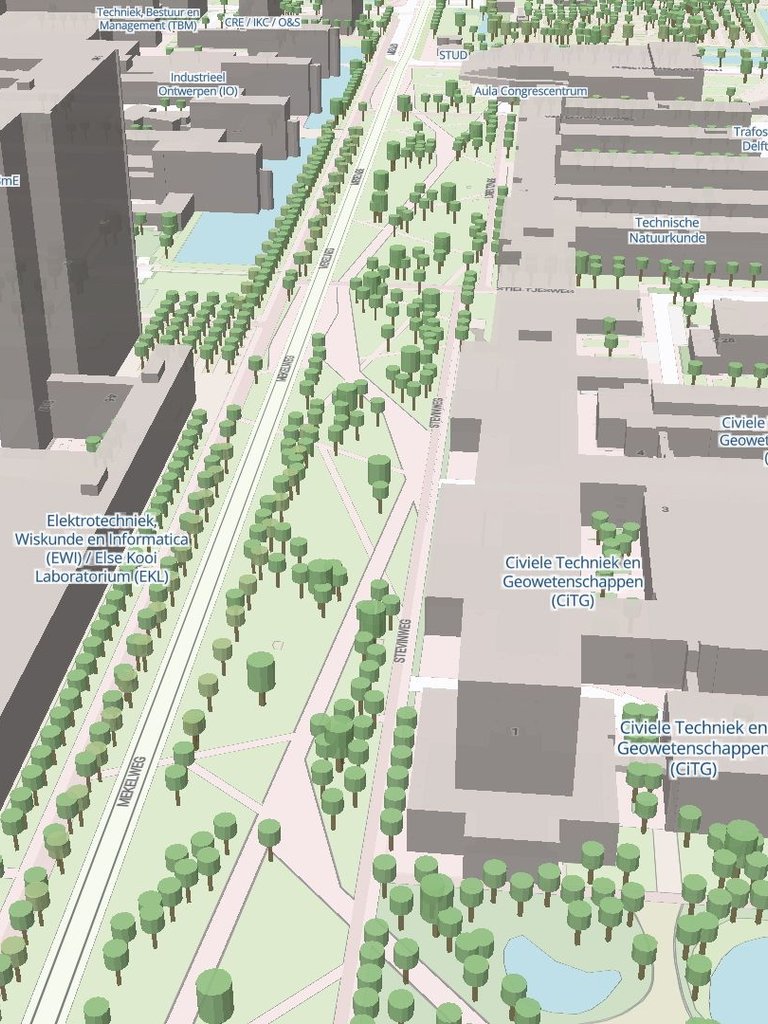
Outdoor mobility dashboard
Interview met Deborah Nas
One of the most important measures against the spread of the coronavirus is social distancing. We are still learning how to put this into practice. There is a lack of insight into how and where people move. Researchers from Delft University of Technology are therefore going to do research into this, starting on their own campus. They hope to gain insight into where it is busy and it is not, and how this changes over time. For example, is there a shift towards cycling, and how is public transport used?
That data comes from sensors, which convert visual information into privacy-proof data. This data is displayed on a dashboard, which becomes a kind of digital twin of the campus: a 3D model with color-coded indications of how busy it is in specific locations. This dashboard serves future scientific research, as the sensor network is intended to grow into a fully-fledged Mobility Lab for scientific research. The project is led by Prof. Serge Hoogendoorn and Dr. Sascha Lanser:
"In addition to the direct applications of the sensor system and the dashboard, there are many and varied possibilities for scientific research. For example, we want to develop new sensor technology with which we can measure more cheaply and reliably. We know from previous research when people experience an indoor or outdoor space as crowded. This so-called level of service has changed with the arrival of COVID-19. It is no longer just about how busy it is on average, but also about how people are spread across a room. The team is now researching how to determine such a new level of service.
An important issue related to this is how we can combine the various data sources as well as possible in order to extract more accurate information from the data (data fusion). Given the variety of data from the various sensors that we use for this research, this is a difficult task, but it is one that we expect will make major leaps in information quality, as well as generate new information. A good example of this is the occupancy rate of the buses that run across the campus. Public transport companies will soon have initial estimates of this occupancy, but we expect to be able to improve these estimates considerably by using artificial intelligence techniques and cleverly combining them with information from our sensors".
Predictions
The ultimate goal is the ability to reliably predict the movements on campus and the locations where we expect problems to occur in the short term (15 minutes) but also in the longer term (days, weeks, months). The data generated by our sensor system offers new possibilities for this. New techniques that we often call artificial intelligence or machine learning lend themselves particularly well to this. We are curious to what extent we can apply such methods, which have already been successfully applied for car traffic, in situations where we are looking at much more complex processes such as walking and cycling flows, but also public transport flows.
We also expect to be able to develop high-quality agent-based models, the combination of which with contamination models is also on the horizon. The data coming from the sensor system, as well as the behavioral insights we can derive from this data, are essential for this. This includes, for example, insights into the behavior of pedestrians and cyclists: how do they behave towards each other and what influence do possibly changed standards have on this? What do people do when they are in busy places? Do they adjust their behaviour and avoid the crowds (by choosing a different route, or not going by bus anymore)? What about the willingness to travel by public transport at all? What effects do measures and advice have on this behaviour? Can we summarize this in mathematical models?
Finally, we want to continue to develop ways to guide and spread the flows on campus in the best possible way, both during and after the period of social distancing. You can think of new algorithms for controlling traffic lights, for example through direct communication with pedestrians and cyclists, offering new transportation services to move to/from and on campus, reservation systems, etc. In short: the possibilities for the use of this research facility are numerous and fit very well with the ambitions of TU Delft: top research with clear societal benefit".