About
Summary of the project
Driving behaviour analytics is an emerging field with new potential for addressing the human factors that are persistently causing a huge burden of traffic injuries. However, there is need for new insights regarding driving profiles and patterns identification and a robust relevant methodology is lacking. The objective of RHAPSODY is to provide evidence for a shift of focus in driving behaviour models, targeting to identify not only the unsafe but also the optimal driving, through the analysis of the dynamic evolution of driving behaviour on both macro- and microscopic levels. Machine learning (ML) and artificial intelligence (AI) techniques will be applied on existing European naturalistic driving data to identify different driver profiles and driving patterns, their rapid changes under different conditions and their variability over individual drivers and populations. Ultimately, RHAPSODY will recognize the benchmarks of optimal driving and investigate the conditions under which drivers may demonstrate best performance. These can be applied for the improvement of safety of both conventional drivers and human-mimic autonomous vehicles (AVs).
Why
Road traffic injuries are a major public health problem in the WHO European Region and cause the premature death of over 80,000 people every year. They are the leading cause of death in young children and adults aged 5 to 29 years. In addition, about 2.7 million people are estimated to be seriously injured annually. Human factors are persistently the main cause of road crashes, with a percentage of 65%–95%. Therefore, it is crucial to understand them in-depth and suggest new approaches to shape safe driving behaviours.
In the current era of naturalistic driving, Big Data availability and advances in modelling techniques, there are considerable opportunities for statistical and AI applications as a basis for driving behaviour analysis, as well as the usage of new data such as driver physiological indicators, variables of driving time and conditions, congestion, road surface and environment conditions, detailed weather and spatial information.
What
The objective of RHAPSODY is to provide evidence for a shift of focus in driving behaviour models, from the unsafe to the optimal driving, through the analysis of the dynamic evolution of driving behaviour in time on both a macro and a microscopic level. The project aims to shed light on the number of different driver profiles and driving patterns that exist, examine whether/how much/how rapidly a driver changes profile under different conditions and reveal how these patterns are differentiated on an individual and driver population level. Insights will also be provided on the prediction of future driver profiles and driving patterns using driving history data. Finally, RHAPSODY will recognize the individual and driver population benchmarks of optimal driving, investigate the conditions under which a driver demonstrates best performance and examine whether they significantly differ among driver profiles.
RHAPSODY will utilize large-scale data currently collected within the ongoing European research project 'i-Dreams'. The i-Dreams project includes datasets coming from a wide spectrum of data sources such as real-time trip recordings of naturalistic driving, real-time physiological indicators of the driver, driving behavioural metrics in either an aggregated form per trip or a disaggregated form of time-series data recordings, as well as indicators for safe driving and questionnaires, recorded from 600 vehicle drivers. Additional spatial data will be sought from open source map providers such as OpenStreetMaps, using APIs.
How
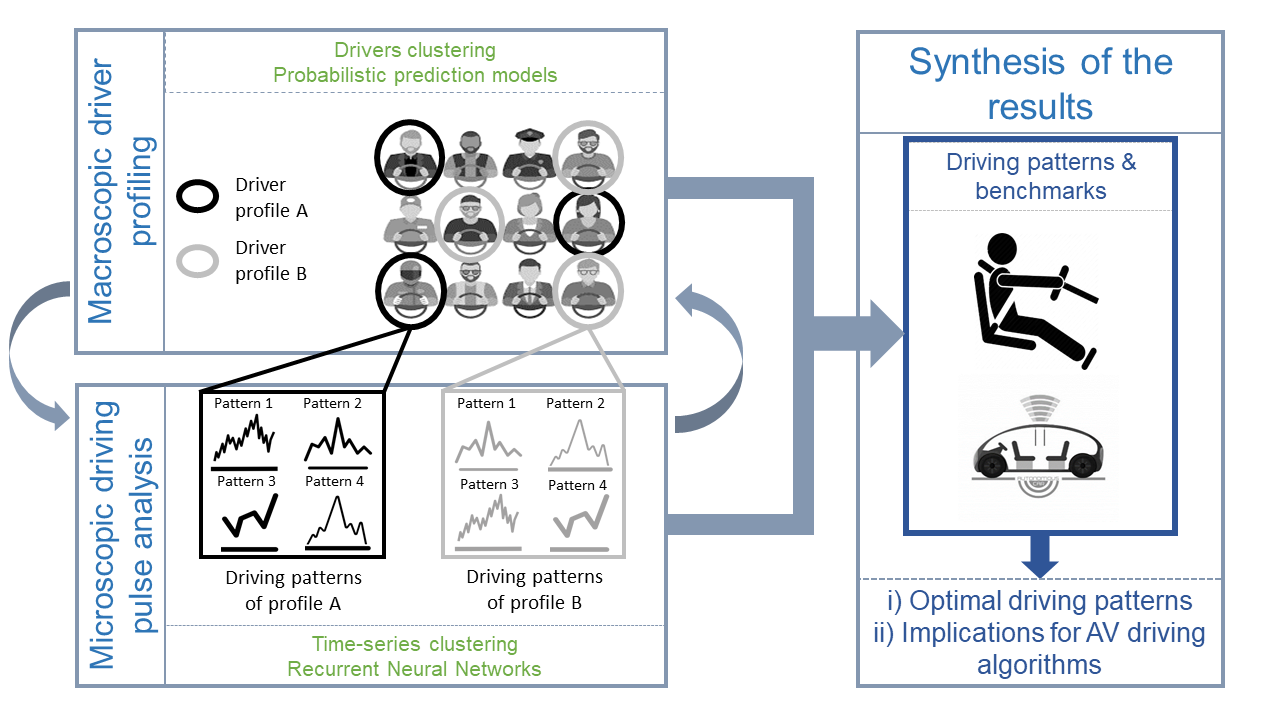
RHAPSODY will analyse driver profiles and driving patterns on two levels shown in the figure above.
On a macroscopic level, ML algorithms will be developed, implemented and tested for driver clustering and profile recognition by exploiting aggregated drivers’ information coming mainly from trip recordings. This part of the study will recognize the existing driver profiles and study in depth the driving patterns identified in each profile, as well as their sequence. The evolution of these profiles in time will also be investigated. On a microscopic level, advanced ML techniques will applied on the driving pulses of each trip to recognize the driving patterns within each driver profile and their association with road risk. Different driving patterns will be distinguished and it will be indicated when, where and under which conditions specific behaviours are present. An algorithm able to understand how driving patterns evolve and provide short-term forecasts of future patterns will be developed.
Integration and synthesis of the results will lead to the comparative assessment of these patterns among drivers of different or the same profile, and among different driving conditions, and eventually to the recognition of the optimal driving benchmarks on an individual level and driver population level. Low risk driving algorithms will be developed aiming to mimic best driving performance. These can potentially also be incorporated in AVs to reduce crash risk.