The Feasibility of Reinforcement Learning as a Replacement for Mixed-Integer Programming for Smart Charging of Electric Vehicles
26 June 2022 20:26 | Add to my calendar
MSc thesis of Simion Becker
This research addresses the feasibility of reinforcement learning as a replacement for mathematical optimization-based smart charging algorithms. This is done through the development of end-to-end Q-learning and Double Deep Q Network reinforcement learning smart charging algorithms. The performance of both algorithms is evaluated on three separate case studies as well as on multiple different random cases and is compared to the charging performance of the average rate charging and mixed-integer programming algorithm benchmarks. As a result, it becomes clear that for individual case studies the Q-learning and DDQN agent are able to find cheap charging moments while charging the vehicle to 100% battery capacity without violating charging constraints. However, when testing the performance of the Q-learning and DDQN agents it becomes clear that the average charging performance is significantly worse than using the method of mixed-integer programming as the algorithms do not learn to generalize well.
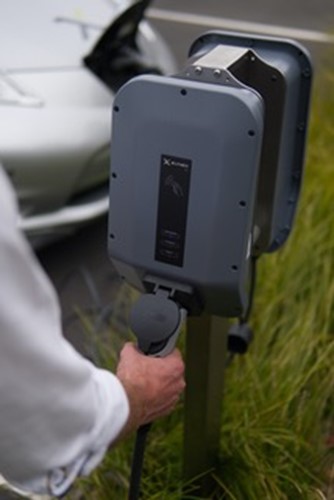