PhD candidates Nail Ibrahimli and Shenglan Du are both part of the 3D Geoinformation group at the faculty of Architecture and the Built Environment. As an example of what AI can do, Nail points out his laptop background. It is a beautiful AI-driven fusion of his holiday photograph of Spanish architecture and a painting by a young artist from Azerbaijan. He often uses the work of artists from his home country to create such mashups, with many appreciating the results. When asked if he is proud of this artwork, or whether he considers himself an artist, Nail laughs self-consciously. “No, I am a programmer. I measure the audience response to my results to improve my models. Art, in my case, is a means to an end.” Read the double interview with two PhD candidates who both work with AI.
What do your academic journeys so far look like?
Nail was born in Azerbaijan but pursued a Bachelor's degree in computer engineering in Turkey before moving to Italy as a research assistant. Parallel to his studies he gained experience working for REscan, a spinoff of the Stanford Research Institute. His Master's degree was in Munich, mainly on image understanding. He became fascinated with digital 3D reconstruction, especially photogrammetry. This process analyses objects based on images from a few different angles.
I contribute to tools which lets architects and students focus on creativity.
Nail Ibrahimli
Meanwhile, Shenglan began with a Bachelor's degree in China focusing on remote sensing, which includes for example satellite and aerial imagery analysis. “The Netherlands drew me in with its English-language programmes, welcoming culture, and affordable living” says Shenglan, who decided to pursue a Master's degree in Geomatics. During her Master she became fascinated by processing point clouds from trees, reconstructing their shapes from raw data. “While a national registry cataloguing almost every Dutch tree is already publicly available, my work provided more precise insights. For example, on their potential to reduce CO2 emissions and impact neighbourhood aesthetics. Without having to manually review each tree.”
Shenglan’s process of modelling trees in four steps. Only the trunk and branches contribute to the point cloud, so her model has to generate the leaves from scratch.
How did you arrive at AI-involved PhD projects?
Shenglan: “I discovered that recent advances in AI made the processing of point clouds faster and more accurate while working in Nijmegen. Now, for my PhD, I am extending the scope to urban objects in general.” She is still in the first phase, training the model using AI and ‘perfect’ point cloud data. The second phase will be evaluating its performance at identifying specific urban objects in real-world measurements. Nail, on the other hand, was initially sceptical of deep learning’s potential for 3D objects but experienced a paradigm shift when he saw demonstrations of self-learning algorithms. For his PhD, he decided to build AI models which combine photogrammetry with artwork analysis to create stylised 3D objects.
Nail’s models can convert pictures to digital 3D objects and simultaneously recolour them based on, for example, paintings. This example was made using his own pictures.
What is the current status of your PhDs?
“For me”, Nail answers, “the project has changed so much since 2020! I need to update my goals almost every month. Papers from more than a few years ago are already outdated, I feel like I am constantly running just to keep up. The advances in OpenAI convinced me to try building a programme which lets you edit 3D objects by simply writing prompts.” Shenglan: “I anticipate that my main challenge will be real-world data’s lower quality and lack of annotation.” Recent advances in AI are not as disruptive for her project as for Nail’s, but she still notices the changes. “The kind of models I use are constantly getting more complex and delivering better results. In the future, we will increasingly rely on semi-supervised or even unsupervised programmes to build our models.”
The kind of models I use are constantly getting more complex and delivering better results.
Shenglan Du
Shenglan aims to build a model which can correctly label urban objects in images. In these examples, the labels of both indoor (left) and outdoor (right) objects are shown in colour.
What makes an AI-based model ‘supervised’?
- Supervised: you give data and corresponding labels, and the model finds the connections between the two.
- Semi-supervised: you give data and some annotations, and the model learns from these sparse instructions and fills in the blanks.
- Unsupervised: you give data and no labels, and the model improves by identifying patterns.
If your projects come to fruition, what will be the impact?
“These models should be able to autonomously identify and label urban objects, eliminating the need for human input and freeing up countless hours of deskwork and fieldwork.” Answers Shenglan.
“I contribute to tools that let architects and students easily generate and edit 3D objects, freeing them from routine tasks to focus on creativity.” says Nail. He thinks the future will bring personalized AI capable of generating content in anyone’s style. He has heard that in ten years, it will be hard to find text that is not AI-generated. And in twenty years, it will be hard to find an image that is not AI-generated. Nail: “If it’s up to me, in thirty or forty years, the same will go for 3D objects.”
Are you worried about the rapid advances in the field of AI?
“I am definitely concerned about the improvement of deepfakes” says Nail. “And I worry about the monopolization of AI by tech giants. Also, we need clearer copyright guidelines for using and reproducing art in AI development.” Shenglan also wonders how AI will reshape the assessment of art and design. “Can we still distinguish diverse designers when everyone is using AI?” And she has many other concerns. “AI has the capability to extract sensitive information from large datasets. The automation of tasks might lead to job displacement. And many researchers are now concerned about the escalating carbon footprint of AI technology.”
Shenglan believes researchers have limited capabilities to address these issues, although she heard that people in the TPM faculty are building frameworks to bridge the gap between AI researchers and policy makers. “Ultimately, it is the government’s job to regulate. I want to see them cooperate with scientists to achieve this goal.” And Nail adds: “Maybe we have to think about creating an independent organisation of scientists and experts to judge developments and potential crises. Sort of like the IAEA, but for Artificial Intelligence.”
More information
- Nail Ibrahimli is doing his PhD research on learning-based 3D reconstruction and 3D urban scene understanding. Shenglan Du her PhD research involves machine learning, 3D geodata analysis, and 3D modelling.
- Shenglan Du and Nail Ibrahimli are both part of the 3D Geoinformation group within the Urbanism department of the Faculty of Architecture and the Built Environment. Both of them work at the 3D Urban Understanding lab.
- Their promotor is Dr. Liangliang Nan.
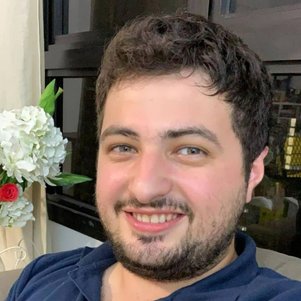
Nail Ibrahimli
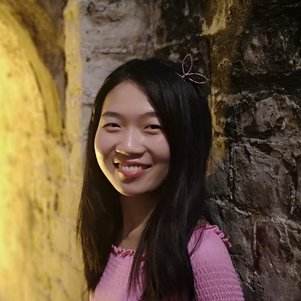