Smart Sensing for Aviation
Smart sensing in aviation consists of three interdisciplinary projects with a cross-faculty team of researchers at TU Delft from SI&C and ATO groups of aerospace engineering department, and CAS and ES groups of EEMCS department. The first of the aforementioned projects focuses on smart ways to use sensors in the manufacturing process in order to improve process efficiency, the second on integrated structural health monitoring sensors to guide efficient maintenance and repair procedures, and the third on developing a method for tracking and understanding passenger movements within airports.
This project works closely with local aviation organisations and industry, including the Schiphol Group, Fokker, Airbus Defence and Space, and others. The project is sponsored by the Ministry of Economic Affairs as a part of the “Topsectoren” policy. The research team has received TKI-funding from the top-sector High Tech Systems and Materials for the Smart Sensing For Aviation Project.
Deep Learning Approach towards Ultrasonic SHM for Smart Aircraft Maintenance
The rise of Internet of Things (IoT) in the recent years, notably in various platforms such as social media (e.g. Facebook), video sharing website (e.g. YouTube), and automatic machine translation (e.g. Google Translate) is primarily contributed by advanced big data processing called deep learning. In these platforms, we witnessed several futuristic features such as facial detection for photo tagging, automated speech recognition, and automatic machine translation, all of which were unimaginable in the previous decades. By seeing many more examples of deep learning applications such as drug discovery, metastatic breast cancer detection, robot vacuum cleaner, and self-driving car, we might agree that deep learning transforms machine learning one step closer into the artificial general intelligence.
Structural Health Monitoring (SHM), as a complement to already existing Non-Destructive Evaluation (NDE) in aerospace and civil engineering, is currently facing many challenges in different aspects such as signal and pattern recognition, system reliability, and environmental noise compensation. Having been shown that deep learning has currently demonstrated a raving success in above mentioned internet platforms, we assume that it is a very promising approach to tackle one of the main challenge in ultrasonic guided wave SHM: automated signal detection and pattern recognition, which also in turns will highly influence the damage detection probability and SHM system reliability.
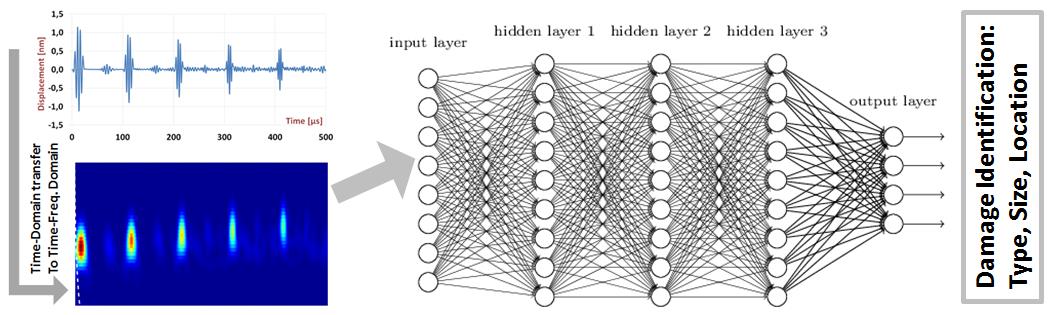
Optical SHM System by FBG Sensors for Smart Aircraft Manufacturing
Fibre Bragg grating (FBG) sensors have proven to be one of the best tools for point internal strain measurements in multilayer materials, namely fibre reinforced composites. Because of the low diameter of the optical fibres, they can be embedded between the layers of the material without sincerely changing its mechanical properties. Under uniform strain variations, the reflected spectra of the FBG sensors will have a strong peak whose wavelength location is linearly proportional to the strain value on the sensor (see image above). However under non-uniform strain distributions, the reflected spectra will get broadened and more complex, and will show multiple smaller peaks at wider range of wavelengths (see image below). In the first part of the project, a few FBG sensors were embedded inside glass fibre composites (see the second figure below), and the specimens were tested under fatigue and static tests. It was shown that using statistical pattern recognition tools and extracting features from the full length of the reflected spectra of FBGs, it’s possible to identify the damage in the vicinity of the sensor, and also classify its type into delamination and matrix crack damages. What we’ll do later in this project is to analyse the complex reflected spectra under non-uniform strains, and try to estimate the strain distribution along the length of the sensor, using global optimisation techniques. The variables of this optimisation problem are in fact the discrete strain variables along the length of the sensor (see photo). Later these strain profiles will be linked to major damage types in composites in order to both identify and classify the damages.
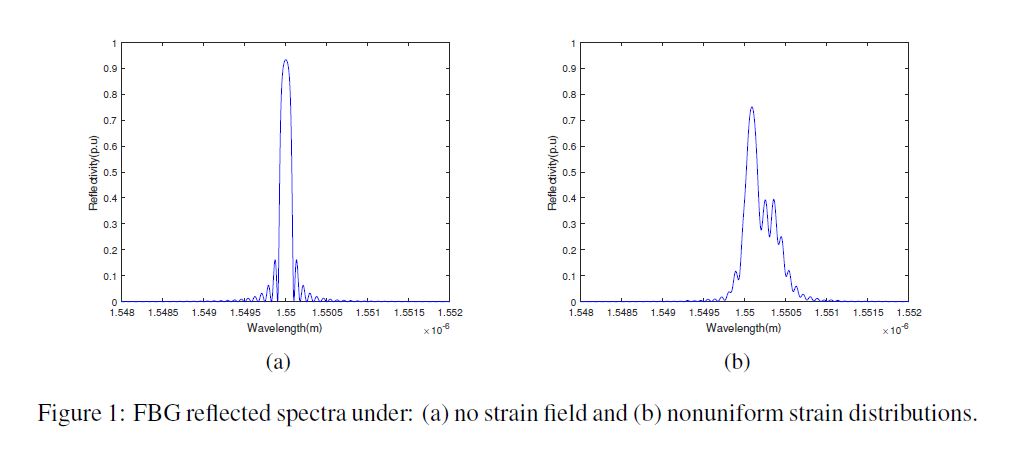
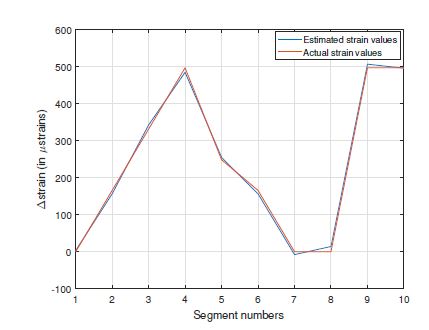
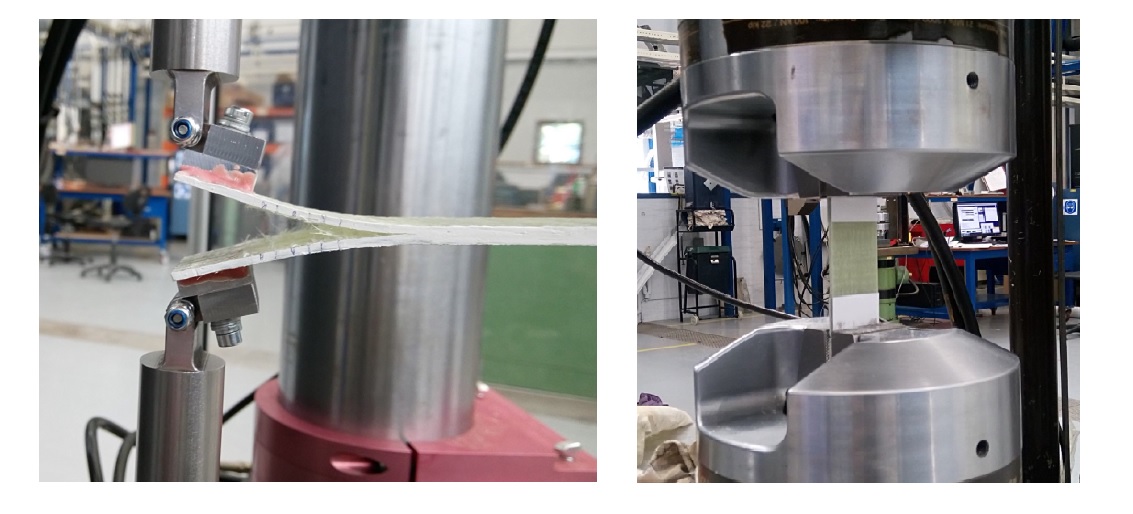