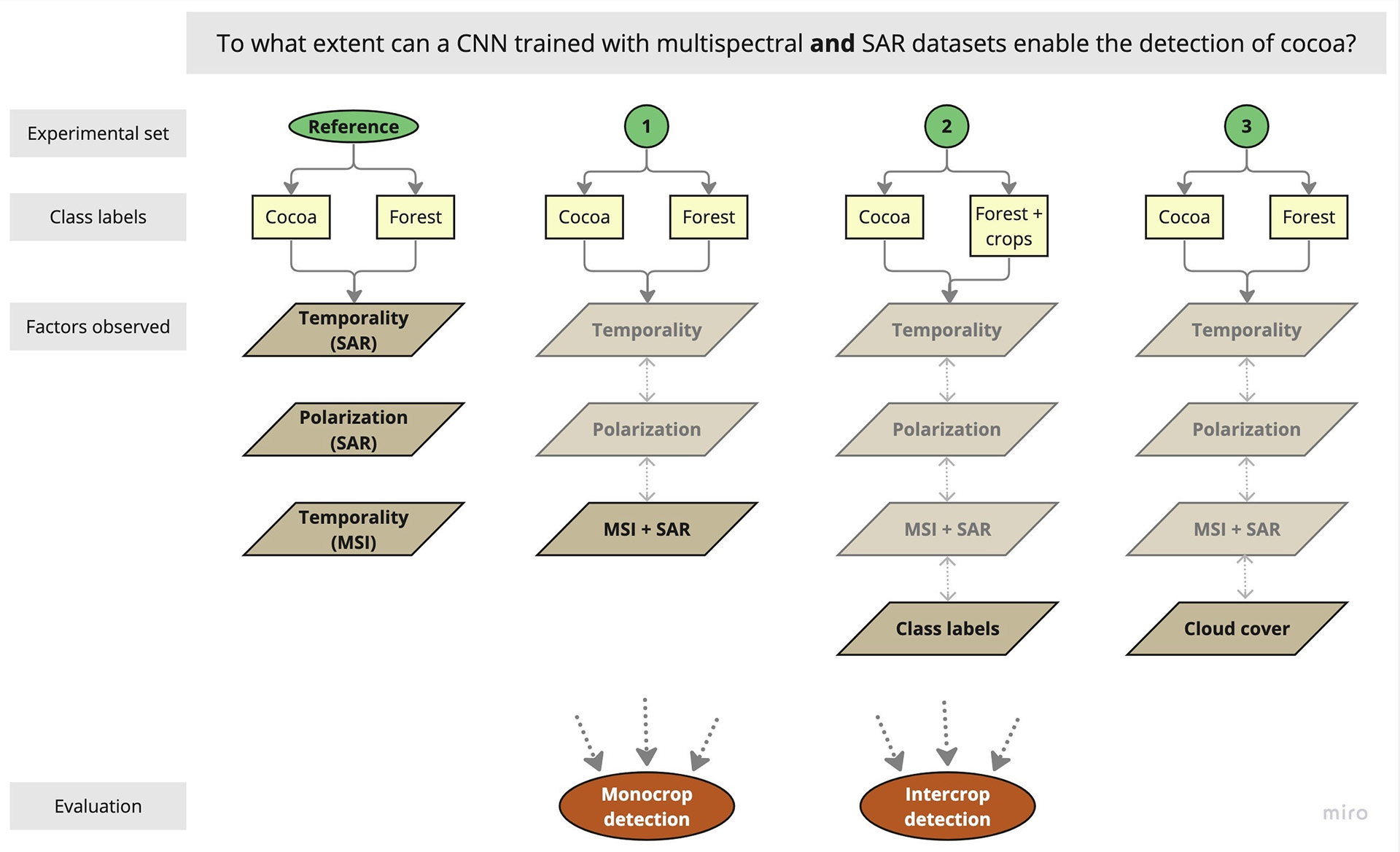
Integrating radar and multi-spectral data to detect cocoa crops: a deep learning approach
The production of cocoa beans contributes to 7.5% of European Union (EU) driven deforestation. For this reason, the recent European Union Deforestation-free Regulation (EUDR) requires producers to perform comprehensive tracking of cocoa farm extents. However, cocoa crops present unique detection challenges due to their complex canopy structure, spectral similarity to forest, variable farming methods, and location in frequently cloudy regions. Previous work employs Multispectral Imagery (MSI) and/or Synthetic Aperture Radar (SAR) for pixel-based classification of satellite images. Convolutional Neural Network (CNN)s offer a promising approach to semantic segmentation of cocoa parcels that considers = both spectral and spatial characteristics. This thesis aims to evaluate the impact of combining SAR and MSI data in the training of a CNN for cocoa detection, in order to demonstrate the importance of texture, moisture and canopy characteristics in identifying cocoa canopies. A U-NET is employed to evaluate
how prediction results are impacted by the stacking of MSI datasets with different SAR polarizations, seasons and temporality. The results show that the addition of single-day and temporal SAR to a single-day MSI image can improve the predictions, reaching an F1 score of 86.62%. This research demonstrates the influence of SAR measurement season and polarization, and ground truth classes, on the semantic segmentation of cocoa.