ALIGN4Energy
Aligning citizens and systems - Combining digital citizen engagement and personalised behavioural interventions to enable system-optimal clean energy investments at scale
Overview
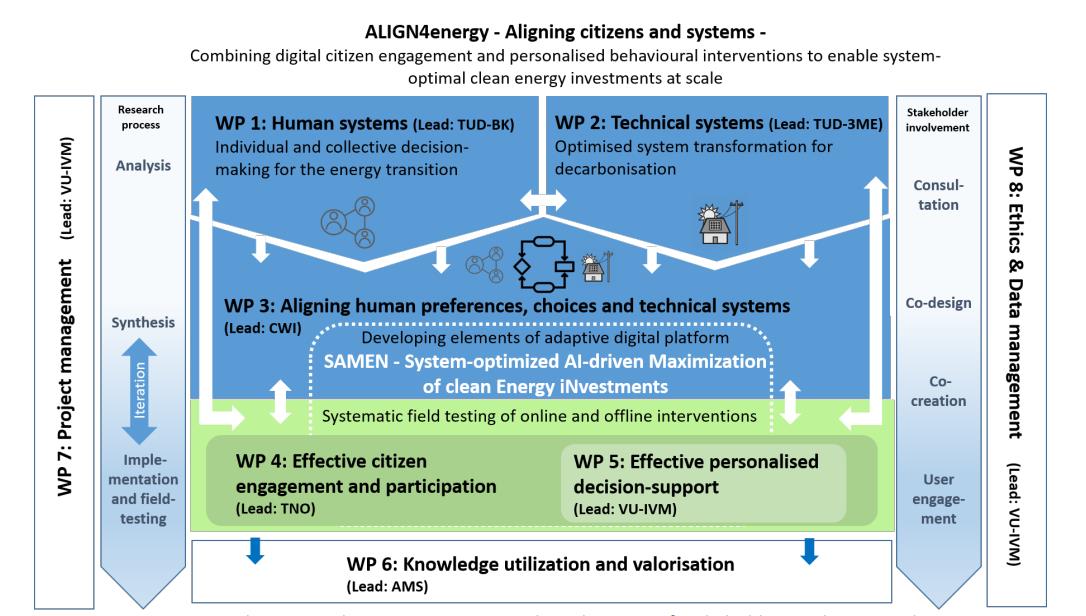
Due to the significant information, coordination, and transaction costs that individuals and citizen collectives face, the energy transition in residential structures takes a very long time. At the same time, policymakers are expected to coordinate a complicated process that determines the overall effectiveness of the new energy system and the cost of decarbonisation. ALIGN4energy aims to address both challenges through eight work packages. It looks at the behavioural, societal and technical sides of this process.
Project Description
This research project aims to resolve the chasm between society’s interest in ensuring our sustained well-being, requiring a swift energy transition, and the lack of progress towards this goal. This requires improving the scientific understanding of human-technical processes, the alignment of human and technical processes, and opportunities for improvements thereof. To this end, we apply and extend state-of-the-art multi-disciplinary research methods in several work packages, in particular aiming for scientific contributions to facilitate a deeper understanding of relevant human decision-making processes (WP1), technical energy system outcome assessments over multiple evaluation criteria (WP2), alignment of technical systems with human objectives (WP3), and intervention designs for engaging citizens (WP4) as well as guiding their decision making in the presence of behavioural barriers and cognitive biases (such as loss aversion, loan aversion or status-quo bias) (WP5). These contributions mutually strengthen one another, with the objective of overcoming the transdisciplinary human-technical challenges inherent to decarbonising the residential sector.
Intended deliverables
• SAMEN platform: activates citizen engagement in retrofitting and provides tailored options that are optimal for citizens, municipalities, and grid operators by calculating the feasibility of each retrofitting
• Load Profile Model: maps individual retrofitting decisions into changes in electricity and heat load profiles.
• Feasibility Calculator: uses mapped load profiles combined with grid constraints to calculate the feasibility of retrofitting options.
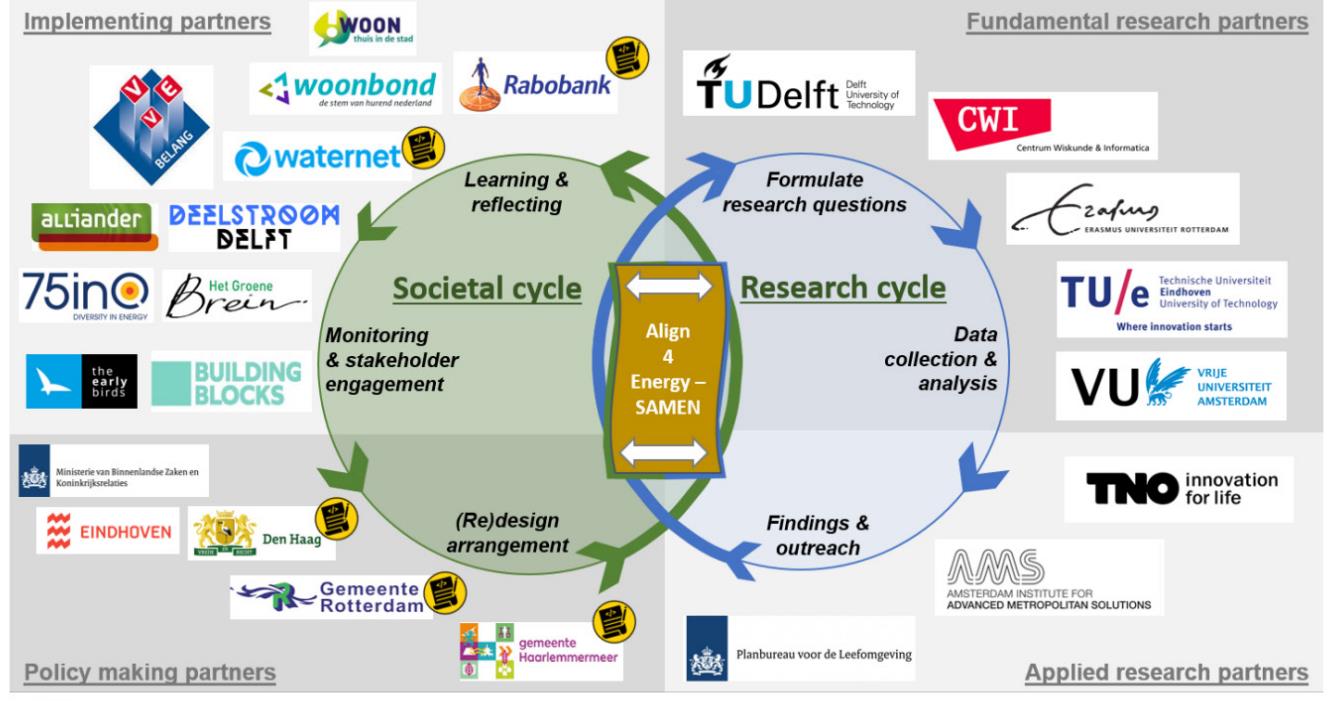
ALIGN4Energy involves partners from different sectors.
Fundamental research partners:
TU Delft (TUD), TU Eindhoven (TUE), Vrije Universiteit Amsterdam (VU), Erasmus University Rotterdam, Centrum Wiskunde & Informatica (CWI)...
Applied research partners:
TNO, AMS Institute, Netherlands Environmental Assessment Agency (Planbureau voor de Leefomgeving)...
Policy-making partners:
Municipality of Eindhoven, Municipality of Den Haag, Municipality of Rotterdam, Municipality of Haarlemmermeer, Ministry of the Interior and Kingdom Relations of Netherlands (Ministerie van Binnenlandse Zaken en Koninkrijksrelaties)...
Implementing partners:
Building Blocks, the Early Birds, Waternet, Rabobank
TUD Team
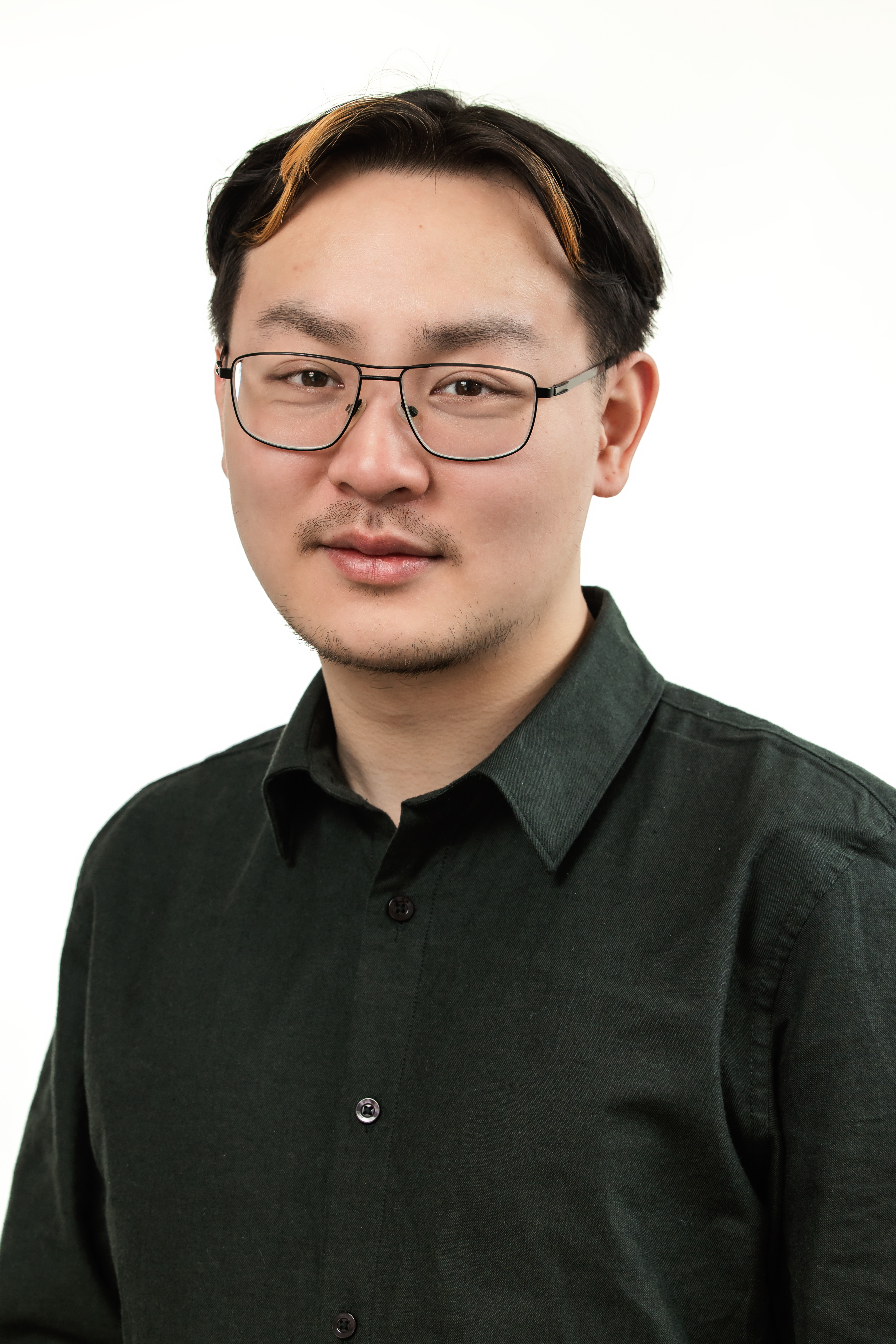
PhD Researcher (work package 2):
N. (Nan) Lin
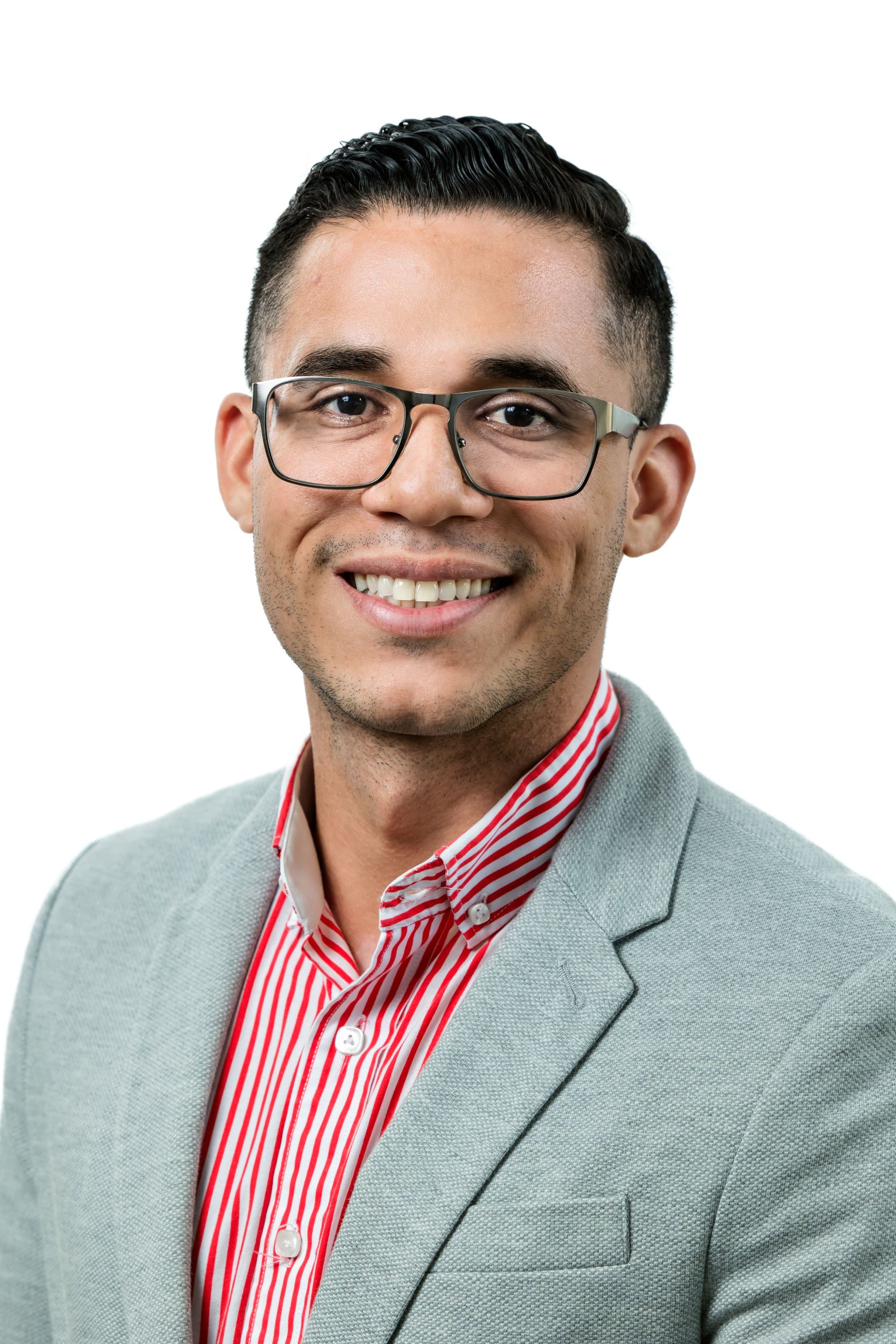
Lead (work package 2):
Dr. P. (Pedro) P. Vergara Barrios
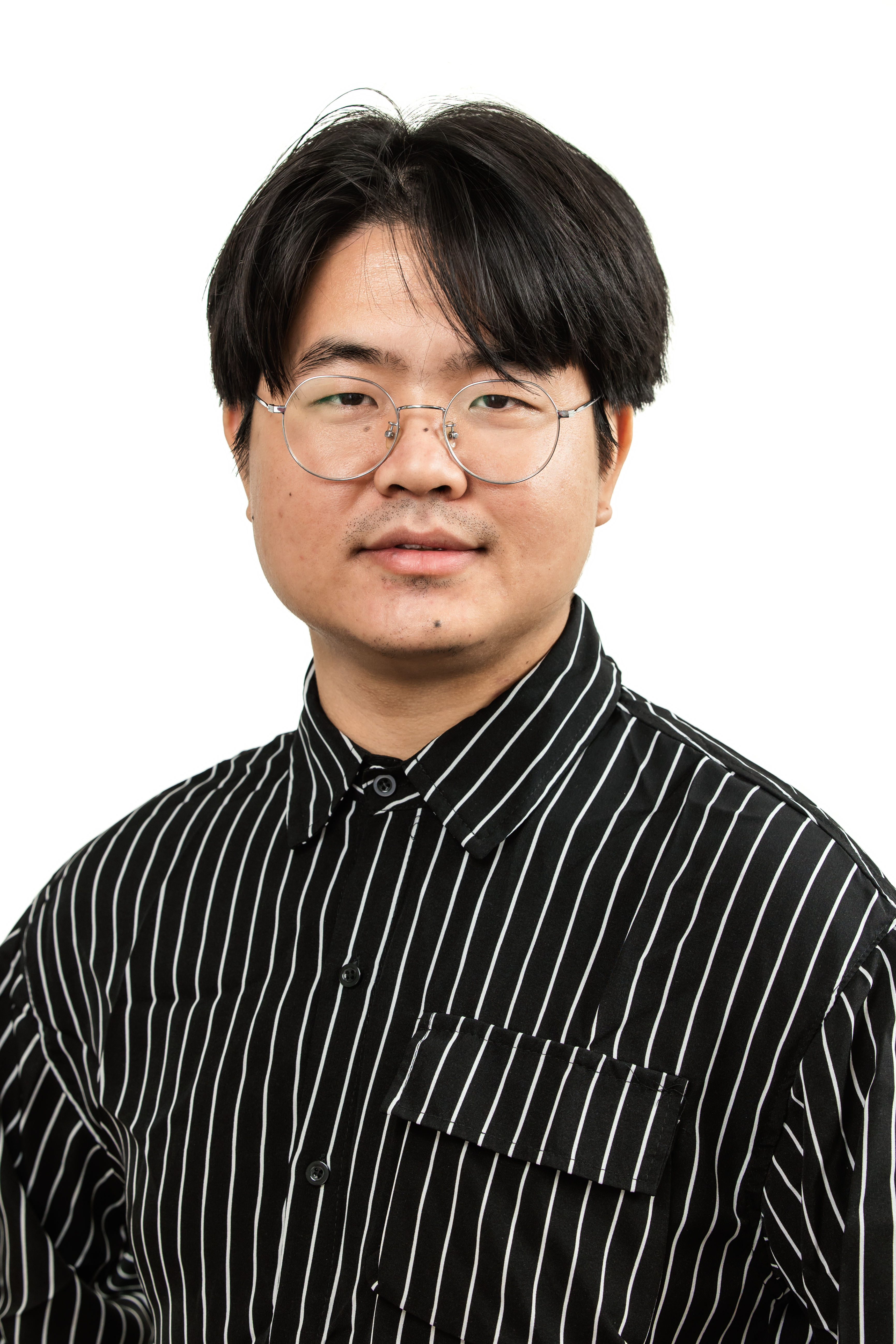
PhD Researcher (work package 2):
W. (Weijie) Xia
N. (Nan) Lin
Nan Lin is a PhD candidate in IEPG Group at Delft University of Technology (TU Delft), the Netherlands. In Aug. 2022, he received his M. Sc. Degree in Electrical Engineering also from TU Delft, in the Signal Processing Systems (formerly known as Circuits and Systems) Group under the supervision of dr. ir. Justin Dauwels and dr. Hadi Jamali-Rad. Generally, his research interests are in machine learning or deep learning from a statistical or probabilistic perspective. More specifically, he is interested in Bayesian learning, copula models, deep generative models, transfer learning, and reinforcement learning. Currently, he is focusing on applications in power systems as his primary job and in computer vision as a side interest.
W. (Weijie) Xia
Weijie Xia is a PhD candidate in IEPG Group at Delft University of Technology (TU Delft), the Netherlands. In Aug. 2022, he finished his master's thesis under the supervision of dr. Phuong H Nguyen in TU/e, and received his M. Sc. Degree in Sustainable Energy Technologies from KTH and TU/e. His research interest is in interdisciplinary areas of machine learning and energy systems. He is also very interested in statistics and related theories.
P. (Pedro) P. Vergara Barrios
Pedro P. Vergara is an assistant professor at the Intelligent Electrical Power Grids (IEPG) group at the Delft University of Technology, in the Netherlands, since October 2020. Before joining TU Delft, he was with the Eindhoven University of Technology, in the Netherlands as a postdoctoral researcher, in which he was involved in several Dutch-funded projects with industry and academic partners. He received his B.Sc. degree (with honors) in electronic engineering from the Universidad Industrial de Santander, Bucaramanga, Colombia, in 2012, and the M.Sc. degree in electrical engineering from the University of Campinas, UNICAMP, Campinas, Brazil, in 2015. In 2019, he received his Ph.D. degree from both institutions, the University of Campinas, UNICAMP, Brazil, and the University of Southern Denmark, SDU, Denmark. Dr. Pedro P. Vergara devotes his research to developing new mathematical programming models and data-driven approaches to operate electrical distribution systems with high penetration of low carbon energy resources (i.e. PVs, EVs, electric heat-pumps, etc).